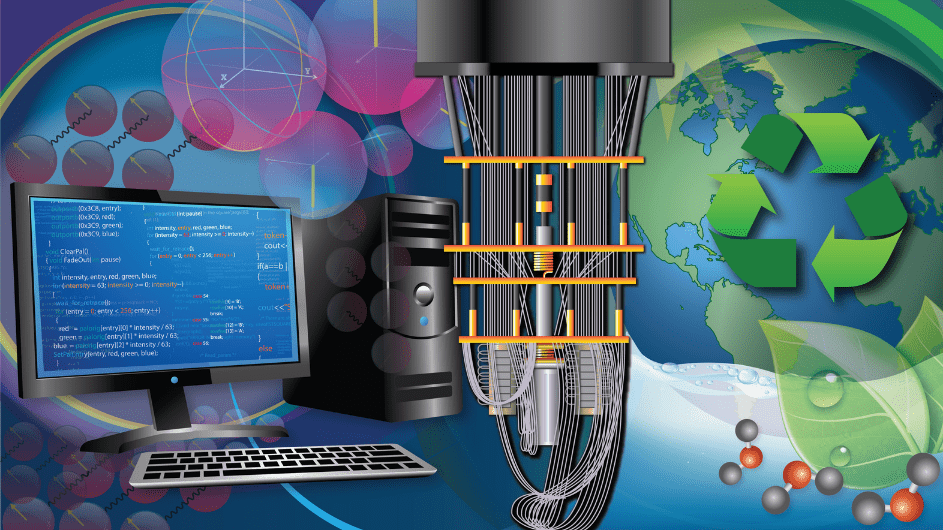
Toward a quantum computer that calculates molecular energy
Researchers have developed an algorithm that uses the most quantum bits to date to calculate ground state energy, the lowest-energy state in a quantum mechanical system. The discovery could make it easier to design new […]