The detection of nuclear spins using individual electron spins has enabled diverse opportunities in quantum sensing and quantum information processing.
Proof-of-principle experiments have demonstrated atomic-scale imaging of nuclear-spin samples and controlled multi-qubit registers. However, to image more complex samples and to realize larger-scale quantum processors, computerized methods that efficiently and automatically characterize spin systems are required.
Scientists have realized a deep learning model for automatic identification of nuclear spins using the electron spin of single Nitrogen-Vacancy (NV) centers in diamond as a sensor.
Based on neural network algorithms, they developed noise recovery procedures and training sequences for highly non-linear spectra. They applied these methods to experimentally demonstrate the fast identification of 31 nuclear spins around a single NV center and accurately determine the hyperfine parameters.
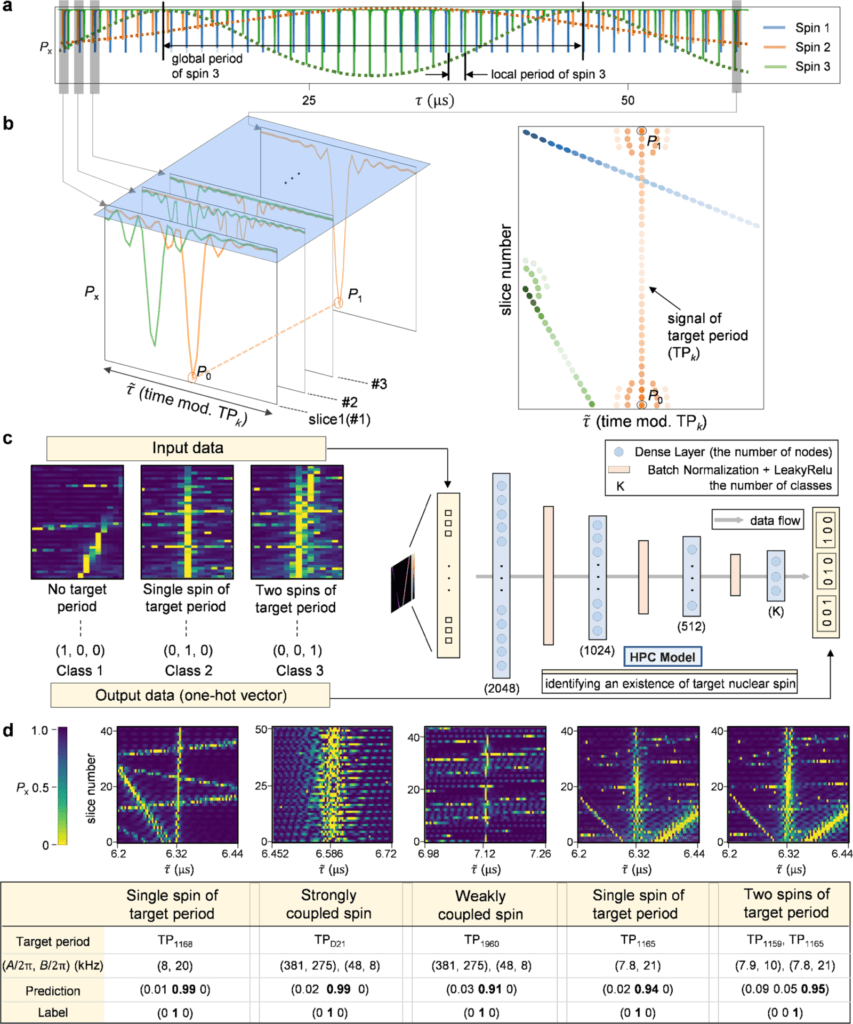
These methods can be extended to larger spin systems and are applicable to a wide range of electron-nuclear interaction strengths.
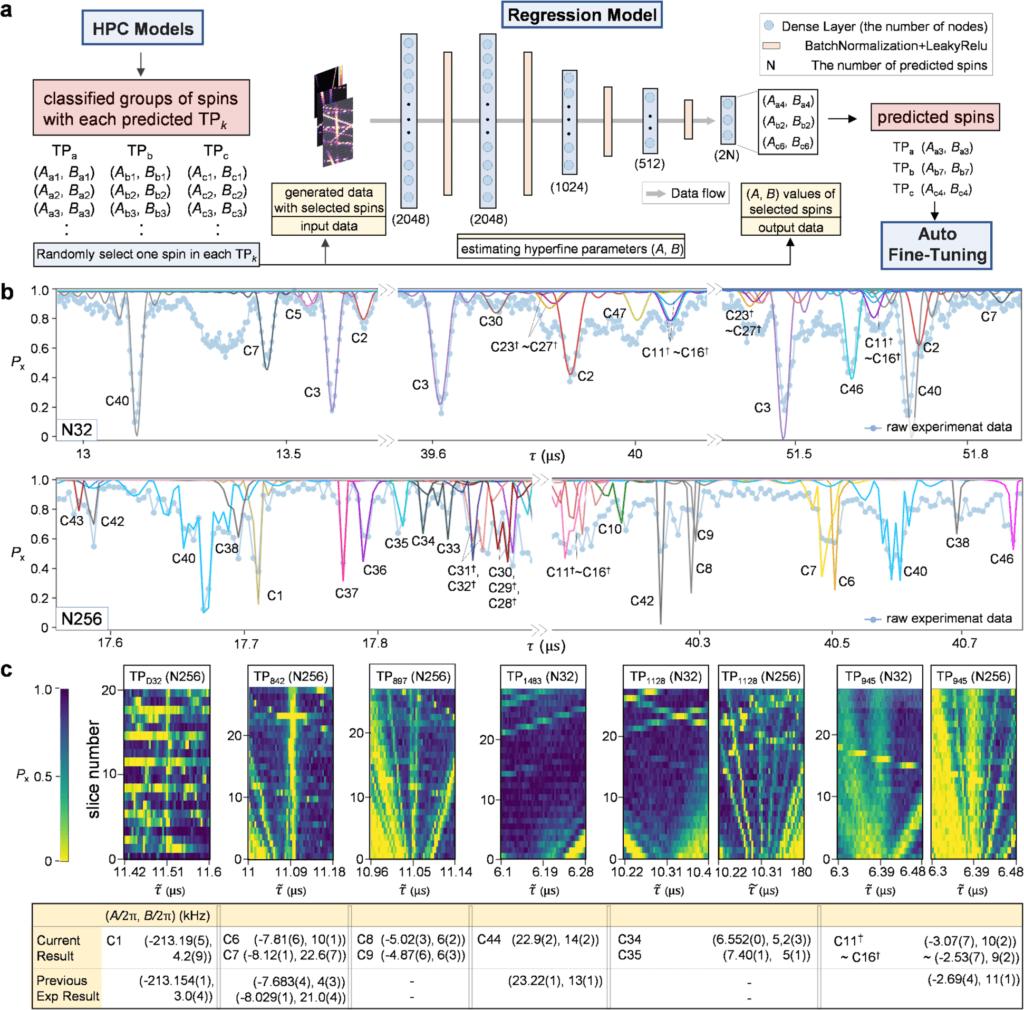
These results pave the way towards efficient imaging of complex spin samples and automatic characterization of large spin-qubit registers.
The paper has been published in npj quantum information.