Cambridge Quantum Computing (CQC) and CERN have posted an article on Arxiv proposing the dual-Parameterized Quantum Circuit (PQC) Generative Adversarial Network (GAN) for generative modelling applications in High-Energy Physics (specifically for generating typical calorimeter images).
The next High Luminosity Large Hadron Collider (HL-LHC) phase will collect an overwhelming amount of data, with complex physics and small statistical error. To analyse this data, high precision methods which use only limited resources are needed. Traditional Monte Carlo based simulation is however very time-consuming, therefore new approaches using deep neural networks have been studied for fast simulations.
GANs are a strong candidate for such fast simulations. Based on two neural networks, generator and discriminator, trained alternatively, GANs have been widely explored thanks to their ability to generate images with complex structures at much high speed. Quantum Generative Adversarial Networks (qGAN) are the quantum version of GANs.
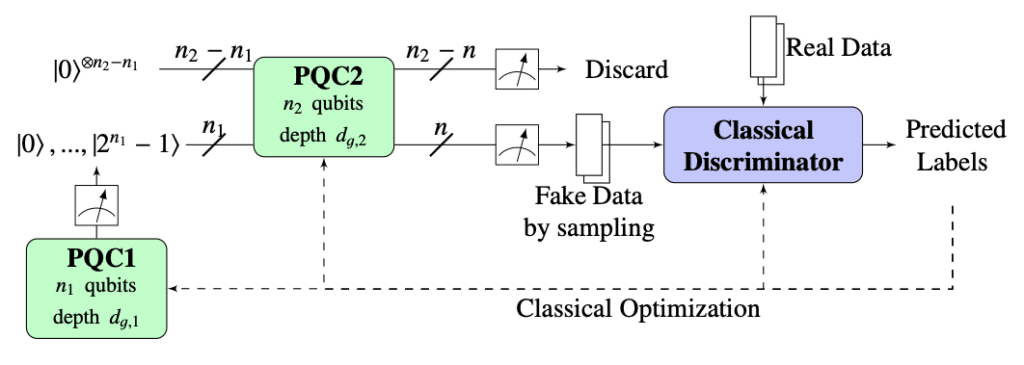
The major innovation of the project was using two PQCs to enable the generation of samples from an ensemble of typical images — something not possible with the conventional qGAN architecture. This model uses two parametrized quantum circuits, which share the role of a single quantum generator: the first PQC learns the distribution over image sam- ples, while the second PQC determines the amplitude distribution over pixels on a single image. Thanks to this separation, it is possible to exploit the continuous nature of probability distributions over output states in quantum circuits to represent continuous variables.
For this reason the team expects the dual-PQC GAN to find application in image processing tasks more generally.