Distributed training across several quantum computers could significantly improve the training time and if we could share the learned model, not the data, it could potentially improve the data privacy as the training would happen where the data is located.
One of the potential schemes to achieve this property is the Federated Learning (FL), which consists of several clients or local nodes learning on their own data and a central node to aggregate the models collected from those local nodes.
However, to the best of knowledge, no work has been done in Quantum Machine Learning (QML) in FL setting yet.
Researchers have presented the federated training on hybrid quantum-classical machine learning models although their framework could be generalized to pure quantum machine learning model.
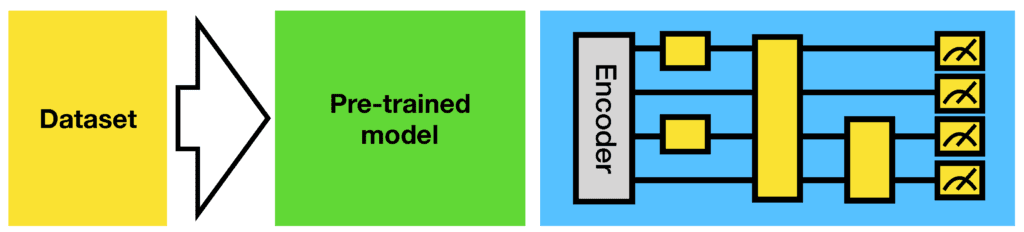
Specifically, they have considered the Quantum Neural Network (QNN) coupled with classical pre-trained convolutional model. Their distributed federated learning scheme demonstrated almost the same level of trained model accuracies and yet significantly faster distributed training. It demonstrates a promising future research direction for scaling and privacy aspects.
The paper has been published in entropy.