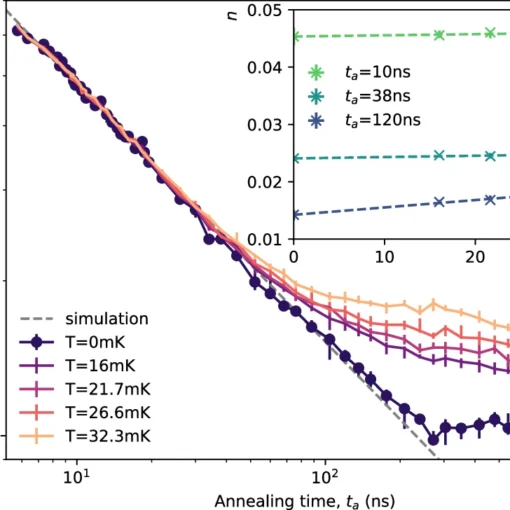
Quantum error mitigation in quantum annealing
Researchers developed practical zero-noise extrapolation techniques for quantum annealing that successfully mitigate both thermal and non-thermal errors in quantum systems without additional qubit overhead, demonstrated through experiments on a transverse-field Ising spin chain that aligned well with theoretical predictions.